Advancing Gen AI-Enabled Asset Reliability at Dow
Exclusive insights from Dow’s Data Science Manager, Senior Data Scientist, and Global Reliability Engineering Discipline Leader
Add bookmark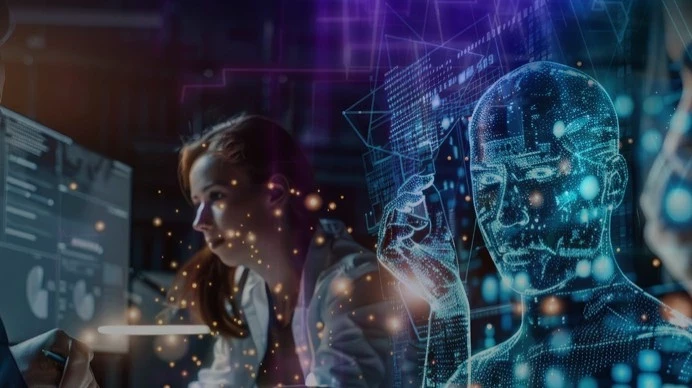
Asset reliability is a critical key performance indicator in any asset-driven business. An IBM study revealed that hybrid AI/ML models, combined with Generative AI (Gen AI), can enable operational teams to prioritize and reduce serial failures by 25%–50% while increasing site reliability by 10%–15%.
Headquartered in Midland, Michigan, The Dow Chemical Company is currently leveraging Gen AI capabilities to automatically extract key failure information that enables the analysis of reliability metrics from multiple sources and sites, including both structured and unstructured data.
Ahead of the AI in Energy Summit in Houston, TX, that took place in February 2024, we spoke with event speakers Swee-Teng Chin, Senior Data Scientist; Peng You, Data Science Manager, Chemometrics and AI and Statistic; and Joaquin Gracia, Global Reliability Engineering Discipline Leader from Dow.
Swee-Teng serves as a senior data scientist in the Chemometrics, AI, and Statistics group, a data science team that supports manufacturing operations. As a senior data scientist and technical leader, her primary responsibilities include leading Gen AI innovation projects within the group, applying analytics in solving plant issues, and ensuring the team has the right expertise to execute technical tasks effectively. She also oversees project selection, resource allocation, and the alignment of efforts within the group.
Peng You, also part of the same data science group, works as a Data Scientist, focusing on implementing and developing data science solutions. This includes building and deploying machine learning models, as well as conducting multivariate analysis for troubleshooting.
Joaquin is responsible for reliability analytics within the company, supporting reliability engineers in adopting and leveraging advanced tools and analytics, such as Artificial Intelligence, to enhance their work and processes.
Read as they share exclusive insights into the development of the Gen AIpowered tool to enhance asset reliability at Dow, and the ongoing validation of this tool.
Oil & Gas IQ: Let’s start by getting a better understanding of the operations of the plant, and the operational challenges it was facing at the time. What was the specific problem you were trying to fix through the Gen AI Asset Reliability Tool?
The current practice of reliability engineers focuses mainly on the analysis of individual or small groups of equipment in each plant for a specific site. One of the key challenges in this process is the highly manual and time-intensive procedure required to align multiple data sources (based on equipment) within a given scope. These data sources include, but are not limited to, global plant maintenance records and assets management data. Another significant complication of this manual alignment procedure lies in the quality of the existing data, as critical maintenance information is often either inaccurately populated or entirely missing.
Oil & Gas IQ: What were you looking for in a Gen AI-enabled asset reliability tool? What tool did you choose and why?
Based on the needs of the end-users, primarily reliability and improvement engineers, we required a highly customized and user-friendly tool designed to provide an integrated platform as the ultimate goal.
Specifically, the tool needed to:
- Allow users to query data from various sources and seamlessly combine it in one place at a global level
- Automatically clean, align, and filter unstructured data for a given scope, potentially leveraging generative AI capabilities
This tool was to serve as an end-to-end solution incorporating data ingestion, preprocessing, and analysis in a single interface and can be easily deployed and updated without causing any interruption to the end user. Additionally, we required an intelligent chatbot enabled by LLM (i.e. enabled by Dow’s own private endpoint of the OpenAI GPT models) to be incorporated with the tool suite for end users to interact with maintenance data directly through natural language.
Oil & Gas IQ: What were the goals/metrics for this project and how far into the project are you now?
This is the first Gen AI Asset Reliability tool that enables users to look at the global maintenance records of all equipment. We are currently in the pilot stage, evaluating the capabilities of LLM and the accuracy of the reliability analysis. As part of the validation metrics, LLM-generated results are then verified against experienced reliability engineers.
Oil & Gas IQ: How does this tool integrate with Dow’s existing systems?
Currently, the primary data source for the tool is SAP, but it can also integrate other data sources. The tool is continuously evolving, with new features being assessed by maintenance and reliability engineers for their suitability in the reliability improvement process. The capabilities of the existing analytics tools extend beyond the scope of the new app.
However, the new app is not intended to anticipate the replacement of any existing global tool; rather, it is designed to complement them.
It will serve as an additional resource within the current Reliability toolbox and will integrate into the existing reliability improvement work process without any modifications. There will be no necessity to alter the current work process.
Additionally, the new app offers more flexibility, better resource optimization for analytics, and includes a chatbot feature to enhance usability.
Oil & Gas IQ: How did you train and validate these AI models given the diversity of equipment and data sources?
At this stage, the tool does not involve training machine learning (ML) or deep learning (DL) models. Instead, it conducts statistical reliability analysis through mostly fitting well-known distributions for specific equipment, rather than relying on global models intended to work across all equipment types. Additionally, pre-trained commercial LLM (i.e. OpenAI’s GPT 4.0 model) is used directly without any additional fine-tuning to perform operations such as content summarization and information extraction on the maintenance records as part of the data processing pipeline. We are validating the results generated by the new tool against experienced reliability engineers in this pilot.
Oil & Gas IQ: Can you share any early results, insights, or impacts on asset reliability since the tool’s deployment along with any unforeseen challenges?
The results we have observed so far are very promising, as they align with the expectations and manual evaluations of reliability engineers. By leveraging this tool, users can save a significant time on querying, cleaning, aligning, and filtering the data required for analysis. Additionally, the users have really appreciated the LLM-enabled chatbot capability, which is a breakthrough as it was not possible in the past to search through tons of maintenance records to find relevant equipment information before Gen AI. As for challenges, we are aware of the limitations of using LLMs, such as their inability to consistently produce errorfree results. To address this, we are incorporating a validation mechanism by experienced reliability engineers to verify the results. Plus, the tool provides users with options to make manual corrections before generating the final reliability model, ensuring greater accuracy and trust in the results.
Oil & Gas IQ: What’s next in terms of rollout to other sites?
This tool is intended for global use, not site-specific, but the concept could be leveraged for other applications.
To learn more about this topic and hear from Dow and other oil and gas operators, join over 350 industry leaders at the Operational Excellence in Oil & Gas Summit, November 4-6, 2025 and hear more about how to explore a smarter, faster, more sustainable path to operational excellence. Learn how leading oil and gas companies have delivered high impact wins on critical priorities — while building long-term momentum through team-driven transformation. This is your opportunity to move from theory into action and align technology, process, and people into a single, cohesive force.