How Can AI Drive Value in Oil and Gas Operations?
A “conversation” with ChatGPT
Add bookmark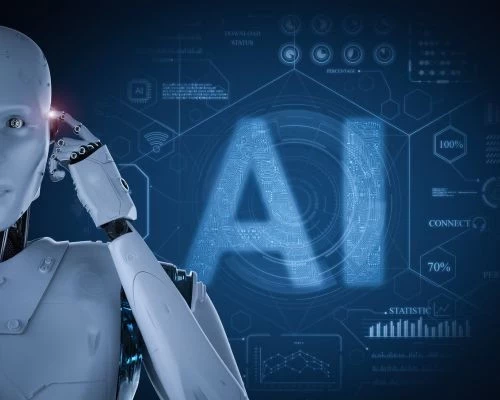
Since ChatGPT exploded on the scene at the end of last year, it has fascinated and terrified in equal measure. The chatbot is a “generative pre-trained transformer,” which in simple terms means that it’s an AI-driven computer program that can hold a well-informed human-like conversation. Many have likened ChatGPT to CP30, a humanoid robot character in the Star Wars series.
So, what does an artificial intelligence-driven bot think about how oil and gas companies can make use of artificial intelligence?
In this “interview,” ChatGPT answers my questions about how oil and gas companies can drive value with AI, structures that best support AI within an organization, and how approaches to data and decision making in the industry need to change to better support it.
While ChatGPT has the mildly annoying habit of responding to all my questions with a numbered list and also has the unfortunate downside of potentially coming up with “plausible sounding but incorrect or nonsensical answers," it otherwise offers up some pretty solid answers. Have a read and see what you think.
Diana Davis, Oil and Gas IQ: How can AI be used in oil and gas?
ChatGPT: AI, or artificial intelligence, has the potential to revolutionize the way that the oil and gas industry operates. Here are some of the ways that AI is already being used in this sector:
1. Predictive maintenance: One of the key benefits of AI is its ability to analyze vast amounts of data and identify patterns and trends that humans might miss. In the oil and gas industry, this can be particularly useful for predicting when equipment is likely to fail and scheduling maintenance before a breakdown occurs. By using AI algorithms to analyze sensor data from equipment like pumps, compressors, and turbines, companies can optimize maintenance schedules and reduce downtime.
2. Remote monitoring: Oil and gas operations are often located in remote and inhospitable environments, making it difficult and expensive for human operators to monitor equipment and processes. AI-powered remote monitoring systems can help bridge this gap by automatically detecting anomalies in data from sensors and alerting operators to potential issues. This can help prevent accidents and equipment failures and reduce the need for on-site inspections.
3. Production optimization: AI can also be used to optimize production in oil and gas fields. By analyzing data from well logs, production reports, and other sources, AI algorithms can help companies identify the most productive zones within a reservoir, predict future production levels, and adjust operations to maximize output while minimizing costs.
4. Exploration and reservoir modeling: AI can also be used to improve the accuracy and efficiency of oil and gas exploration and reservoir modeling. By analyzing seismic data and other geologic data, AI algorithms can help geologists and engineers identify the most promising areas for exploration and estimate the size and shape of oil and gas reservoirs.
5. Safety and risk management: Finally, AI can be used to improve safety and risk management in the oil and gas industry. By analyzing data from sensors and other sources, AI algorithms can help identify potential safety hazards, predict the likelihood of accidents or spills, and recommend mitigation measures to reduce risk. This can help companies comply with safety regulations and prevent costly accidents and environmental damage.
Diana Davis, Oil and Gas IQ: What are the challenges that oil and gas companies have in implementing AI?
ChatGPT: While AI has the potential to bring significant benefits to the oil and gas industry, there are also several challenges that companies face in implementing these technologies. Here are a few of the main challenges:
1. Data quality: AI algorithms rely on high-quality data to generate accurate insights and predictions. In the oil and gas industry, however, data can be messy, incomplete, or inconsistent, particularly when it comes from legacy systems. This can make it difficult for AI algorithms to generate reliable results. Companies must therefore invest in data quality assurance processes and data governance frameworks to ensure that the data they use is fit for purpose.
2. Data integration: Oil and gas companies generate vast amounts of data from a variety of sources, including sensors, production reports, and geological surveys. To get the most out of AI, companies must be able to integrate and analyze this data in a meaningful way. This requires a robust data architecture that can handle large volumes of data from disparate sources.
3. Skill shortage: AI requires a specific set of skills, including data science, machine learning, and programming, which can be in short supply in the oil and gas industry. Companies must therefore invest in training programs to develop these skills in their existing workforce or recruit new talent with the necessary expertise.
4. Cost: Implementing AI can be expensive, particularly in the early stages of adoption when companies may need to invest in new hardware and software infrastructure, as well as hiring skilled personnel. Companies must therefore carefully weigh the costs and benefits of AI adoption and develop a clear business case before committing to major investments.
5. Cultural change: Finally, implementing AI requires a cultural change within organizations. AI requires a different way of thinking about data and decision-making, which can be difficult to adopt in traditional oil and gas companies with hierarchical structures and conservative cultures.
Companies must therefore invest in change management processes to ensure that employees are comfortable with the new technologies and are able to integrate them into their workflows.
Diana Davis, Oil and Gas IQ: In what ways does thinking about data and decision-making need to change?
ChatGPT: Adopting AI can require a significant shift in the way that oil and gas companies think about data and decision-making. Here are a few of the ways that this shift might manifest:
1. Data-driven decision-making: In traditional oil and gas operations, decisions are often made based on experience, intuition, and rules of thumb. AI requires a more data-driven approach, where decisions are based on insights generated by analyzing vast amounts of data. This means that companies must be willing to challenge traditional ways of working and embrace a more evidence-based approach to decision-making.
2. Interdisciplinary collaboration: AI requires expertise from a range of disciplines, including data science, engineering, and geology. This means that oil and gas companies must break down silos between different departments and encourage interdisciplinary collaboration. By bringing together experts from different fields, companies can generate more comprehensive insights and make more informed decisions.
3. Agile and iterative processes: AI requires a flexible and iterative approach to data analysis and modeling. This means that companies must be willing to experiment with different approaches and adjust their methods based on feedback from the data. This requires a culture of continuous improvement and a willingness to learn from failures.
4. Risk management: AI can generate new insights and identify new opportunities, but it can also introduce new risks. Companies must therefore develop robust risk management frameworks to ensure that they are able to manage potential risks and mitigate them before they become significant issues.
5. Real-time decision-making: AI can generate insights in real-time, which can be particularly useful in operations where decisions need to be made quickly. This means that companies must be prepared to make decisions quickly based on real-time data and be able to adjust operations as circumstances change. This requires a flexible and agile organizational structure that is able to respond quickly to changing conditions.
Diana Davis, Oil and Gas IQ: What kind of organizational structures support AI?
ChatGPT: Organizational structures that support AI typically have the following characteristics:
1. Cross-functional teams: AI requires expertise from a range of disciplines, including data science, engineering, and domain-specific knowledge such as geology or reservoir engineering. Cross-functional teams bring together experts from different fields to work collaboratively on AI projects, which can lead to more comprehensive insights and more effective decision-making.
2. Agile methodologies: AI projects require a flexible and iterative approach to development, which is best supported by agile methodologies. Agile methodologies prioritize collaboration, communication, and continuous improvement, which are all important for successful AI projects.
3. Decentralized decision-making: AI can generate insights in real-time, which requires decentralized decision-making structures that empower employees to make decisions quickly based on real-time data. Decentralized decision-making structures can also help to reduce bottlenecks and enable faster innovation.
4. Data-centric cultures: AI relies on high-quality data to generate accurate insights and predictions. Organizations that are data-centric prioritize the collection, analysis, and management of data, and have processes in place to ensure data quality and integrity.
5. Continuous learning: AI is a rapidly evolving field, and organizations that are able to adapt and learn quickly are more likely to succeed in implementing AI. Continuous learning can take many forms, including training programs, knowledge sharing sessions, and experimentation with new technologies and approaches.
Overall, organizations that are able to break down silos between different departments, prioritize collaboration and communication, and empower employees to make data-driven decisions are more likely to succeed in implementing AI.
Diana Davis, Oil and Gas IQ: What advice would you give oil and gas professionals on how to drive value with AI?
ChatGPT: Here are a few pieces of advice for oil and gas professionals looking to drive value with AI:
1. Identify high-impact use cases: AI has the potential to transform many aspects of the oil and gas industry, but not all use cases are equally valuable. To get the most out of AI, it's important to identify high-impact use cases that can generate significant value for the organization. These might include optimizing production, reducing downtime, or improving safety.
2. Start small and scale up: Implementing AI can be a complex and challenging process, and it's important to start small and scale up gradually. This allows organizations to experiment with different approaches, test their assumptions, and learn from their mistakes before committing to major investments.
3. Invest in data quality: AI algorithms rely on high-quality data to generate accurate insights and predictions. To drive value with AI, oil and gas professionals must therefore invest in data quality processes to ensure that the data they use is fit for purpose.
4. Collaborate with experts: AI requires expertise from a range of disciplines, including data science, engineering, and domain-specific knowledge such as geology or reservoir engineering. To drive value with AI, it's important to collaborate with experts from these fields to ensure that AI solutions are based on sound technical and scientific principles.
5. Develop a clear business case: AI can be expensive to implement, and it's important to develop a clear business case before committing to major investments. This requires a thorough understanding of the potential benefits and risks of AI, as well as a realistic assessment of the costs involved.
6. Prioritize change management: AI requires a cultural change within organizations, and it's important to prioritize change management to ensure that employees are comfortable with the new technologies and are able to integrate them into their workflows.
Overall, oil and gas professionals looking to drive value with AI should focus on identifying high-impact use cases, starting small and scaling up, investing in data quality, collaborating with experts, developing a clear business case, and prioritizing change management.
Diana Davis, Oil and Gas IQ: Thank you for your time!
ChatGPT: You're welcome! If you have any more questions in the future, feel free to ask.
What do our human readers think? Does ChatGPT get it right?